Note: A fab (short for fabrication plant) is a highly specialized factory where semiconductor chips are manufactured in ultra-clean environments. You’ll see this term used throughout the blog to refer to these production facilities.
For decades, semiconductor manufacturing has been a game of precision—an industry where even the tiniest imperfection can lead to massive production failures and revenue losses. Today, manufacturers can’t afford inefficiencies, with the demand for next-gen chips skyrocketing across sectors like IoT and high-performance computing.
From wafer to chip, the semiconductor manufacturing process is an intricate choreography of precision engineering, with hundreds of steps spanning weeks. And with rising demands for faster, smaller, and more energy-efficient chips, the pressure of fabs has never been higher. Yields must go up; defects must go down – and there’s zero room for error.
This is precisely where AI steps in, not as a helper but as a game-changer.
Data and Gen AI services in semiconductor fabrication is changing the game by redefining how chips are produced and is significantly revolutionizing semiconductor manufacturing by automating processes, enhancing precision, and optimizing production workflows.
By leveraging AI-powered semiconductor production, chipmakers are achieving flawless defect detection and unprecedented yield optimization. There is no more guesswork—just smarter, faster, and more efficient semiconductor manufacturing.
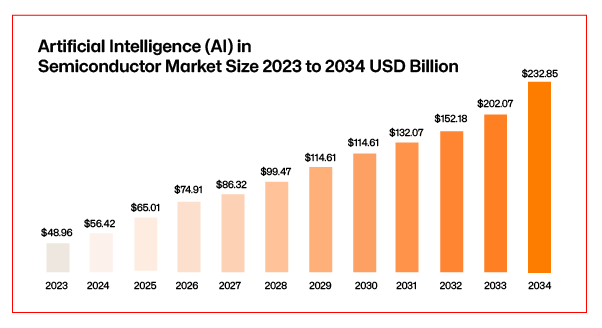
In this blog, we will explore how AI-driven defect detection, yield optimization, and predictive analytics are transforming semiconductor fabrication, enabling manufacturers to enhance efficiency, streamline resource usage, and accelerate next-gen chip production.
The Hurdle of Microscopic Defect Detection
One of the most significant challenges in semiconductor fabrication is identifying minuscule defects that can disrupt chip performance. Each chip begins its journey as a silicon wafer and undergoes a series of processes—oxidation, deposition, photolithography, etching, ion implantation, and more. These processes are repeated dozens of times to create transistors and interconnects, layer by layer, often numbering in billions. As chip architectures shrink to 5nm, 3nm, and beyond, even a single misplaced atom can lead to device failure, performance degradation, or low yield rates.
Traditional automated optical inspection (AOI) systems rely on rule-based algorithms that often struggle with:
- Inconsistent detection accuracy, missing subtle pattern variations
- High false-positive rates, leading to unnecessary rework
- Limited adaptability, failing to recognize new defect types as manufacturing processes evolve.
Maintaining high yields and reducing defects has involved:
- Manual inspection and classification
- Statistical Process Control (SPC)
- Engineer-driven root cause analysis
- Reactive quality control
However, these methods are no longer sufficient. Even aided by traditional tools, the human eye can’t keep up with the precision and speed demanded today. That’s precisely where AI begins to shine – in automating, accelerating, and amplifying every part of the process.
The Increasing Demand for Optimization in the Semiconductor Sector
AI is now at the center of semiconductor production, powering smarter, faster, and more efficient operations. From anticipating defects to optimizing resource allocation, AI allows companies to increase yield, reduce downtime, and shave operational costs. One of the most powerful tools, Generative AI, is transforming workflows—eliminating waste, speeding design iterations, and raising production efficiency.
What once started as semiconductor-powered technology has now come back full circle—AI is, in turn, shaping the semiconductor industry itself. As algorithm complexity increases and data requirements skyrocket, AI’s role has shifted from an ancillary feature to an underlying pillar. It’s now intricately a part of R&D, production processes, and even marketing itself, building an extremely potent feedback loop that’s driving innovation anew every day.
In today’s high-stakes world, AI integration is not only a competitive advantage—it’s essential. Semiconductor firms that adopt AI-based optimization are not only catching up with industry expectations; they’re shaping the future standard.
Ready to scale semiconductor efficiency? Discover how AI drives defect-free, high-yield fabrication!
Explore Service
Why AI? A Look at the Semiconductor Industry’s Pain Points
The semiconductor sector is the quiet driver of the digital age—spurring innovations through smartphones, laptops, automotive technologies, industrial machines, and others. Its transition from the earliest transistor in the mid-20th century to the ultra-small, high-performance chips of today has been driven by insatiable technological progress and rampant demand for electronic products.
The industry’s exponential growth path, dominated for years by Moore’s Law, has yielded higher processing performance, lower cost per function, and incremental device miniaturization. This advancement has been achieved through innovations in manufacturing processes, including photolithography, ion implantation, chemical vapor deposition, etching, and new semiconductor materials that enable faster, smaller, and more energy-efficient devices.
However, despite its tremendous advancement, the industry faces a new age of challenges across multiple dimensions—from design to logistics, manufacturing, and data optimization. Overcoming these barriers is critical to maintaining innovation and unlocking the next level of semiconductor development.
1. Chip Design, Technology, and Innovation Challenges
One of the most pressing concerns is growing chip design complexity and exploding fabricating costs. As technology node sizes shrink, manufacturing gets progressively more complicated—requiring atomic-scale precision and testing conventional tools to their extremes.
Newer technologies like AI, IoT, and 5G demand even more powerful, specialized chips, putting tremendous stress on semiconductor players to innovate as fast as possible. Survival depends on staying competitive, keeping enormous R&D expenditures, and cultivating a strong workforce capable of implementing cutting-edge solutions into current systems.
This high-pressure game continuously challenges players to satisfy simultaneous demands for innovation, performance, energy efficiency, and manufacturability.
2. Supply Chain and Logistics Challenges
The semiconductor supply ecosystem is inherently global, with materials, components, and know-how distributed worldwide. This interconnectedness begets logistical weakness, which is magnified in periods of disruptive disturbances such as the COVID-19 pandemic or periods of geopolitical conflict.
Procurement, inventory, and distribution management across international suppliers is a high-risk, high-complexity problem. A single bottleneck at one location in the supply chain can propagate throughout the entire manufacturing line, impacting timeliness and dollars.
Resilience through diversification, visibility in real-time, and predictive analytics is a strategic imperative.
3. Multi-Step Production and Yield Optimization
Semiconductor production is a multi-step process involving wafer growth, fabrication, packaging, and testing. Each process is intricate, extremely sensitive, and vital to output quality.
As device geometries decrease, precision demands become more stringent. Sustaining high yield and quality through thousands of micro-scale production variables is technically demanding and costly. Even minor process variations can result in higher defect rates and wasted material.
In addition, global demand variations tend to cause excess production or idle capacity, affecting profitability and supply chain effectiveness. Yield improvement strategies, process control techniques, and lean manufacturing are key to overcoming this hurdle.
4. Data Gathering and Process Improvement
Semiconductor manufacturing is increasingly based on data intelligence with each wafer, tool, and test producing gigantic amounts of data. However, that also implies that controlling and interpreting this data is becoming a task.
Sophisticated data-gathering and analysis systems are now critical to help maximize operations. With the power of data from fabrication equipment, metrology stations, and test systems, manufacturers can:
Track Equipment Health: Real-time information identifies anomalies and forecasts failures before shutting down production.
Apply Statistical Process Control (SPC): Pattern detection and control charts help ensure processes are stable and within specs.
Facilitate Quality Assurance: Detection of defects early on minimizes waste, rework, and expense while ensuring consistent product quality.
Now, let’s dive into how AI solves these challenges in real time.
How AI is Reshaping the Semiconductor Manufacturing
Combining AI and machine learning further amplifies these capabilities, allowing for predictive analytics, intelligent automation, and accelerated decision-making throughout production.
Wafer manufacturing is among the most complex and challenging steps in semiconductor production, comprising several hundred narrowly controlled operations. Historically, process optimization relied largely on iterative testing and mechanical fine-tuning, a process that no longer meets the needs of the rapidly increasing complexity of advanced node technology.
To overcome today’s challenges and improve performance, semiconductor firms are adopting digital transformation—driven by AI to redefine the manufacturing environment. From predictive analytics to real-time monitoring, AI allows manufacturers to drive efficiency, lower costs, and improve quality at every step of wafer fabrication.
Predictive Analytics for Process Optimization
AI is revolutionizing wafer manufacturing through predictive analytics. Using past production data, AI models can predict inefficiencies, possible defects, and yield loss ahead of time. This pre-emptive optimization of process parameters reduces trial-and-error, lowers production errors, and maximizes overall yield.
Example: AI in deposition and etching (PECVD, RIE, Metallization) enables systems to dynamically control deposition and etching rates in real-time. This guarantees uniform film thickness and reduces micro-defects, which is essential for device performance and reliability.
Real-Time Process Monitoring and Control
AI technologies are transforming real-time process control. AI algorithms constantly process IoT sensor data embedded in fabrication equipment to identify anomalies. The system instantly adjusts, keeping the process within optimal parameters. This transition from reactive to autonomous process control minimizes error propagation and greatly enhances throughput, particularly in high-volume production settings.
Revolutionizing Quality Assurance with AI
Traditional defect detection has been time-consuming, highly operator-dependent, and manual. Shift-to-shift variations frequently lead to missed defects or variable quality control.
AI-powered systems now enable manufacturers to inspect more wafers at much higher speeds—without sacrificing accuracy. This revolution eliminates bottlenecks, improves product reliability, and reduces time-to-market for new semiconductor products.
Predictive Maintenance and Equipment Uptime
Unexpected equipment downtime is one of the key cost drivers in wafer fabs. Predictive maintenance systems using artificial intelligence analyze tool health constantly based on sensor data, picking up early indicators of wear or failure.
This enables maintenance teams to plan interventions within non-critical production windows, maximizing uptime and prolonging equipment life.
Optimizing Raw Material Use and Strengthening the Supply Chain
Wafer manufacturing is based on costly raw materials—silicon wafers, specialty gases, chemicals, and photoresists. AI reduces material wastage by analyzing production data and optimizing consumption patterns.
In addition, AI facilitates intelligent inventory management and demand planning, enabling timely procurement and avoiding production delays and their associated costs. This enhances supply chain resilience and simplifies end-to-end operations.
Yield Optimization and Cost Efficiency
Yield loss may be due to machine uptime, wafer handling, contamination, and process inefficiencies. Based on cross-functional datasets, AI systems can determine patterns, root causes, and interdependencies among these variables.
By doing so, manufacturers can initiate specific process enhancements that directly boost yield. Even a slight increase in yield can mean substantial cost savings and added profitability.
Speeding Innovation in R&D
The miniaturization of semiconductor devices has increased research and development efforts and costs. AI assists R&D teams in simulating, prototyping, and verifying new concepts at unprecedented speeds.
AI-based modeling and simulation tools provide rich insights into material science, process interactions, and performance behavior. Such tools have played a crucial role in developing next-generation materials such as graphene and carbon nanotubes, creating new avenues of semiconductor performance.
Conquering the Miniaturization Challenges
As the industry moves toward sub-3nm technologies, accuracy is everything. EUV lithography is now aided by AI models that offset optical aberrations and enhance pattern accuracy.
AI also helps maximize heat dissipation in dense chip architectures, keeping performance and reliability on track despite increasing power densities. These tools play a critical role in providing functional chips at atomic-scale dimensions.
Now, this is not just theory. Let’s uncover the success story of how TSMC turned to AI to revolutionize defect detection and slash defect rates by 40%!
Winning with AI: TSMC’s Journey to a 40% Reduction in Defect Rates
Taiwan Semiconductor Manufacturing Company (TSMC), the world’s largest semiconductor foundry, faced increasing defect rates in its advanced 7nm and 5nm fabrication lines. Traditional defect detection methods could not keep up with the sheer complexity of these nanoscale architectures.
The AI Solution
TSMC implemented a deep learning-powered defect detection system trained on billions of wafer images, allowing it to identify and classify defects with 95% accuracy.
Results
- 40% reduction in defect rates across its advanced node production lines
- 20% improvement in overall chip yield, leading to increased production efficiency
- Millions saved annually by reducing material waste and production delays
By integrating AI in semiconductor defect detection, TSMC maintained its leadership in high-performance chip manufacturing, ensuring that only the highest-quality chips reached the market.
Not just TSMC – semiconductor giants like Intel, Samsung, and more have also implemented AI to optimize yield, enhance quality control, and accelerate time-to-market.
Intel
Intel uses deep learning models to detect defects in die images with accuracy exceeding that of human inspectors. Their AI systems reduce defect escape rates and optimize mask inspection time.
Samsung
Samsung applies AI to improve photoresist coating uniformity and optimize plasma etching – critical steps in 3nm node manufacturing. These aren’t experimental pilots. These are production-scale deployments transforming billion-dollar operations.
AI is reshaping semiconductor manufacturing—ready to join the shift?
Get in touch
Final Thoughts: AI’s Role in Next-Gen Chip Manufacturing
AI is no longer an add-on in semiconductor manufacturing—it’s the backbone of precision, efficiency, and yield optimization. From AI-driven defect detection to smart manufacturing for semiconductors, the industry is undergoing a massive shift.
With every defect detected before it becomes a problem and every fabrication process optimized to squeeze out the highest yield possible, AI is redefining semiconductor production as we know it.
The question isn’t whether manufacturers should adopt AI. The real question is – can they afford not to?
Are you looking to implement AI in your fab or manufacturing operations? Whether you’re building ML pipelines, defect classification systems, or predictive maintenance models, now is the time to harness AI’s full power with Indium. Let’s co-create the fab of the future—smarter, faster, and flawlessly efficient.